Getting Started¶
System Requirements¶
MC3
(version 1.2) is known to work on Unix/Linux (Ubuntu)
and OSX (10.9+) machines, with the following software:
- Python (version 2.7)
- Numpy (version 1.8.2+)
- Scipy (version 0.13.3+)
- Matplotlib (version 1.3.1+)
- mpi4py (version 1.3.1+)
- Message Passing Interface, MPI (MPICH preferred)
MC3
may work with previous versions of these software;
however, we do not guarantee nor provide support for that.
Install¶
To obtain the latest MCcubed code, clone the repository to your local
machine with the following terminal commands.
First, keep track of the folder where you are putting MC3
:
topdir=`pwd`
git clone https://github.com/pcubillos/MCcubed
Compile¶
Compile the C-extensions of the package:
cd $topdir/MCcubed/
make
To remove the program binaries, execute (from the respective directories):
make clean
Example 1 (Interactive)¶
The following example (demo01) shows a basic MCMC run with MC3
from
the Python interpreter.
This example fits a quadratic polynomial curve to a dataset.
First create a folder to run the example (alternatively, run the example
from any location, but adjust the paths of the Python script):
cd $topdir
mkdir run01
cd run01
Now start a Python interactive session. This script imports the necesary modules, creates a noisy dataset, and runs the MCMC:
import sys
import numpy as np
import matplotlib.pyplot as plt
sys.path.append("../MCcubed/")
import MCcubed as mc3
# Get function to model (and sample):
sys.path.append("../MCcubed/examples/models/")
from quadratic import quad
# Create a synthetic dataset:
x = np.linspace(0, 10, 100) # Independent model variable
p0 = 3, -2.4, 0.5 # True-underlying model parameters
y = quad(p0, x) # Noiseless model
uncert = np.sqrt(np.abs(y)) # Data points uncertainty
error = np.random.normal(0, uncert) # Noise for the data
data = y + error # Noisy data set
# Fit the quad polynomial coefficients:
params = np.array([ 20.0, -2.0, 0.1]) # Initial guess of fitting params.
# Run the MCMC:
posterior, bestp = mc3.mcmc(data, uncert, func=quad, indparams=[x],
params=params, numit=3e4, burnin=100)
Outputs¶
That’s it, now let’s see the results. MC3
will print out to screen a
progress report every 10% of the MCMC run, showing the time, number of
times a parameter tried to go beyond the boundaries, the current
best-fitting values, and corresponding \(\chi^{2}\); for example:
::::::::::::::::::::::::::::::::::::::::::::::::::::::::::::::::::::::
Multi-Core Markov-Chain Monte Carlo (MC3).
Version 1.2.0.
Copyright (c) 2015-2016 Patricio Cubillos and collaborators.
MC3 is open-source software under the MIT license (see LICENSE).
::::::::::::::::::::::::::::::::::::::::::::::::::::::::::::::::::::::
Start MCMC chains (Fri Feb 5 10:45:17 2016)
[: ] 10.0% completed (Fri Feb 5 10:45:17 2016)
Out-of-bound Trials:
[0 0 0]
Best Parameters: (chisq=111.0541)
[ 3.79473869 -2.73050517 0.51636233]
...
[::::::::::] 100.0% completed (Fri Feb 5 10:45:18 2016)
Out-of-bound Trials:
[0 0 0]
Best Parameters: (chisq=111.0449)
[ 3.77284276 -2.72330815 0.51634107]
Fin, MCMC Summary:
------------------
Burned in iterations per chain: 100
Number of iterations per chain: 3000
MCMC sample size: 29000
Acceptance rate: 39.04%
Best-fit params Uncertainties S/N Sample Mean Note
3.7728428e+00 3.8407332e-01 9.82 3.7694995e+00
-2.7233081e+00 2.1964109e-01 12.40 -2.7232216e+00
5.1634107e-01 2.6891868e-02 19.20 5.1641806e-01
Best-parameter's chi-squared: 111.0449
Bayesian Information Criterion: 124.8604
Reduced chi-squared: 1.1448
Standard deviation of residuals: 2.93518
At the end of the MCMC run, MC3
displays a summary of the MCMC sample,
best-fitting parameters, uncertainties, mean values, and other statistics.
Note
More information will be displayed, depending on the MCMC configuration (see the Tutorial).
Additionally, the user has the option to generate several plots of the MCMC sample: the best-fitting model and data curves, parameter traces, and marginal and pair-wise posteriors (these plots can also be generated automatically with the MCMC run). The plots sub-package provides the plotting functions:
# Plot best-fitting model and binned data:
mc3.plots.modelfit(data, uncert, x, y, title="Best-fitting Model",
savefile="quad_bestfit.png")
# Plot trace plot:
parname = ["constant", "linear", "quadratic"]
mc3.plots.trace(posterior, title="Fitting-parameter Trace Plots",
parname=parname, savefile="quad_trace.png")
# Plot pairwise posteriors:
mc3.plots.pairwise(posterior, title="Pairwise posteriors", parname=parname,
savefile="quad_pairwise.png")
# Plot marginal posterior histograms:
mc3.plots.histogram(posterior, title="Marginal posterior histograms",
parname=parname, savefile="quad_hist.png")



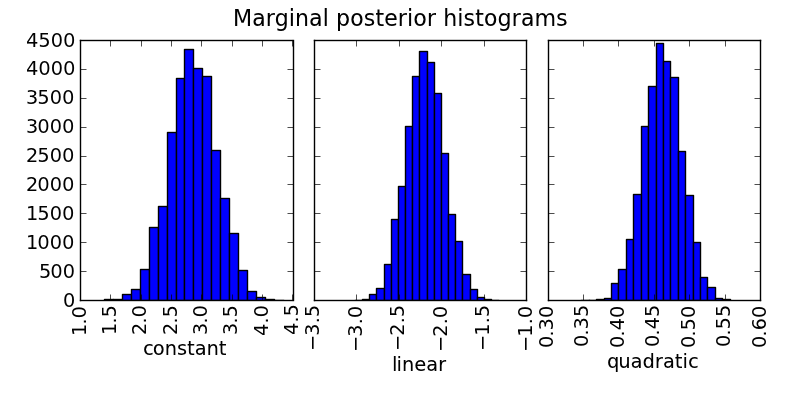
Note
These plots can also be automatically generated along with the MCMC run (see File Outputs).
Example 2 (Shell Run)¶
The following example (demo02) shows a basic MCMC run from the shell prompt. To start, create a working directory to place the files and execute the program:
cd $topdir
mkdir run02
cd run02
Copy the demo files to run MC3
(configuration and data files):
cp $topdir/MCcubed/examples/demo02/* .
Call the MC3
executable, providing the configuration file as
command-line argument:
mpirun $topdir/MCcubed/MCcubed/mccubed.py -c MCMC.cfg
Note
If you don’t have MPI or dont want to use it, make the previous call as:
python $topdir/MCcubed/MCcubed/mccubed.py -c MCMC.cfg
Noting that, in this last case, you need to have mpi=False
.